AI’s uses continue to expand, from medical and finance to urban planning, wildlife monitoring, and even reconstructing the periodic table of elements. This growing emphasis on AI — and its applications to all business models — has resulted in a significant surge in demand for personnel who can design and operate with this new technology.
As a result of increasing demand, firms are now struggling to find qualified candidates for AI roles. In fact, it takes more than 100 days on average to fill AI-related positions. Demand pressure (the ratio of employment demand to talent availability) has climbed to over 30% in places like the Bay Area, Seattle, and New York. It’s no wonder, however, that 54% of CIOs trying to use AI cite staffing as the most difficult obstacle.
To better locate and employ AI talent (and other important people in today’s digital environment), organizations’ hiring procedures and tactics must evolve.
One of the most important adjustments the greatest recruiting firms are making is the use of analytics, and they need your assistance as a talent analytics specialist to do so.
In the last six months, our TalentNeuron team has reviewed over 500 AI-related job descriptions for various firms to learn what talents organizations are seeking for and where they are searching. We’ve uncovered a few insights into how talent analytics experts might collaborate with their recruitment teams to hire essential, hard-to-find talent using AI positions as an example.
Widening the Candidate Pool Through Skill Analysis
Our first result, based on the job descriptions we examined, is that firms’ AI-related job descriptions are convoluted and unrealistic. Companies, for example, have been searching for AI expertise with extra capabilities in Internet of Things, image processing, and other areas. Such skill requirements considerably limit the potential pool, yet most recruitment teams (or, at the very least, their hiring managers) have no understanding what that talent pool looks like or what the repercussions of these sophisticated skill needs are. In most circumstances, looking for applicants that meet even 80% of the skill criteria would lengthen the hiring process.
Data may be useful in broadening hiring managers’ perspectives on applicant quality and assisting them in adjusting their skill needs. So, what information do hiring managers and recruiters want from you?
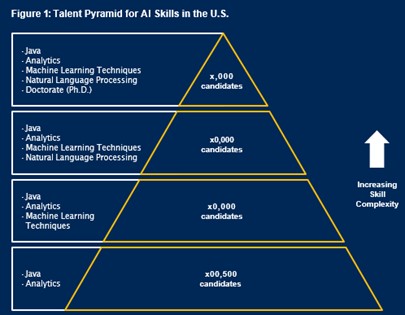
Taking geographic location as an example, places like the Bay Area, New York, and Seattle have reached a saturation point for AI and machine learning engineers, but talent analytics can identify alternative areas with moderate supply and demand for AI talent (see Figure 2). Recruiting in these locations will help organizations capture the market early and lessen the challenge of salary costs. The emerging hubs could reach maturity in the next three to five years, depending on ecosystem growth and AI adaptation.
Another alternative source for AI talent is university collaboration; many organizations are partnering with universities in the form of scholarships, internships, or fellowship programs to source AI engineers. Other organizations consider gaining access to highly skilled AI talent by acquiring the startups they are currently working with or via platforms that provide competition among AI, machine learning, or data scientist communities. Kaggle (owned by Google) is one such platform that offers a machine learning competition and a cloud-based workbench for data science and AI.
Talent analytics can be used to evaluate the quality of candidates from these alternative sources and compare them with candidates from typical sources.
Conclusion and Recommendations
These strategies can be used to help source and hire hard-to-find talent beyond AI. By evaluating job requirements and talent sources, talent analytics professionals can give recruiters a more accurate understanding of what their organizations must do to get the talent they need.
As you partner with your recruiting team, we recommend these action steps:
- Prioritize business-aligned data. When collecting data on hard-to-find talent, be sure to measure what the business and hiring managers need to know, not just what is nice to know. Understand their priorities and how they like to make decisions.
- Explain the “so what.” Defining metrics and terminology is not as powerful as communicating why each metric or term matters to recruiters and the business.
- Remember why recruiters and hiring managers need the data you are presenting and make the consequences of not acting clear.
- Think visually, not just analytically. Designing a compelling story will make it easier to convince recruiters and hiring managers to look elsewhere for the talent they need, and relying on visuals, not just numbers, can help develop this story.