Centralization has dominated classic scientific, social, and economic developments. Decentralization has also received increasing attention in management, decision, governance, and economics, despite its incomparability in AI.
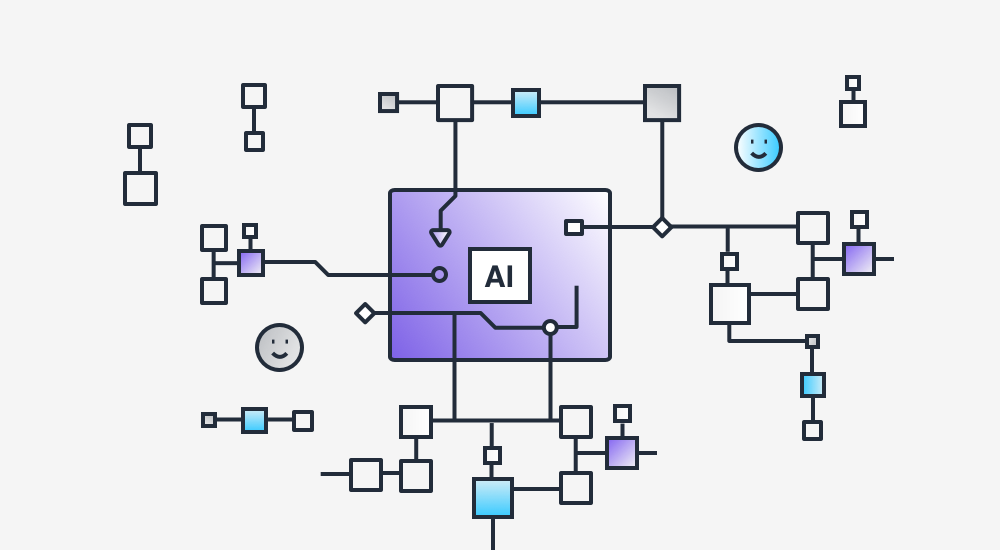
Decentralization complements and enhances centralization for systematic, all-round, and multifold objectives, functionality, and consequences. The paradigm shift from centralization to distribution has substantially mitigated methodology, knowledge, and capability gaps in social science, management science, decision science, economics, computing, complex systems, and AI.
On the other hand, open-source and science have made paramount complementary achievements and contributions; blockchain is transforming and inspiring finance to decentralized finance (DeFi) and the World Wide Web to Web3. In contrast, decentralizing AI is still an open area.
By reviewing decentralized movements, systems, and technologies, including the recent movements on the blockchain, Web3, and decentralized science (DeSci), we will dig into decentralized AI (DeAI), in edge intelligence and the tasks of synthesizing centralized AI (CeAI) and DeAI. These further envisage and inspire the opportunities of DeAI-enabled smart blockchain, Web3, metaverse, and DeSci.
Distributed to Decentralized Systems
In #technology, the thinking and conceptualization of technological decentralization have evolved for over half a century. A flagship decentralized IT movement is the open and shareable initiatives and programs, represented by open source, open data, open access and open science.
A representative early-stage #distributed and decentralized computing flagship is the formation of Linux operating systems and their ecosystems. This movement has been further intensified by public and private cloud services for decentralized infrastructures, storage, sharing, computing, applications (DApps) and services.
In IT practices, a recent trend is that an increasing number of organizations have removed or are decentralizing their existing centralized IT divisions, substituted by subscribing to vendor-centralized cloud-based infrastructures, computing, applications and services.
Furthermore, edge computing emerges to connect isolated end-level devices and the Internet of Things (IoT) through edge nodes (networks) rather than centralized cloud infrastructures and to support decentralized computation, communication, storage, sharing and management at end devices or edge nodes.
Blockchain and Web3 further decouple the dependence on and interactions with central or intermediary authentication, organization, computation, communication, and mediation. Decentralized operations, authentication, communication, and collaborations engage scalable decoupled and loosely connected individuals and local nodes to form decentralized ecosystems physically, virtually, mentally, or jointly, resulting in evolving blockchain, Web3, the metaverse and DeSci movements.
Why DeAI?
From Centralized to DAI and DeAI: Conventional AI aims, technologies, systems and services are centralized, static, predefined at design time and user neutral. CeAI presents its outputs as either a standalone system or a multiagent system.
When a multiagent system carries the AI task, CeAI usually has a central controller, mediator, matchmaker, coordinator, or communicator (for message passing) to manage and govern agents and task execution. CeAI met with fundamental issues, such as inflexibility, high vulnerability, poor reliability, and low scalability and adaptability, to complex real-world demand and large-scale problem-solving.
The CeAI thinking does not fit the individualized nature and personalized demand/supply in the physical and social worlds. DAI then emerged, followed by DeAI to further flatten DAI.
Distributed AI involves multiagent systems for parallel or distributed problem-solving.
A DAI system decomposes a problem or task into subproblems or sub-tasks and then assigns them to specialized agents for handling. The agent-based partial solutions are then synergized to form the solution to the problem.
While taking a bottom-up approach, DAI usually involves mediation, cooperation, coordination, and communication between agents for task decomposition, assignment, execution, and consensus-building. Orchestration and consensus-building mechanisms further consolidate distributed agents, logic, tasks and results for solution aggregation or formation.
Therefore, DAI is not truly decentralized, nor the classic parallel and distributed computing.
The emergence of DeAI addresses the thinking and technological gaps in CeAI and DAI for genuine AI decentralization, including decentralizing multi-agents, AI tasks, task execution, resource allocation, storage and management, transaction management, intelligence management and consensus-building between decentralized agents and AI tasks.
On one hand, DeAI promotes the evolution and development of AI toward individualized, personalized, distributed, secure, privacy-preserving intelligence and intelligent problem-solving. On the other hand, DeAI also aims to incorporate intelligence and intelligent problem-solving into the existing decentralized systems and their enabling platforms, such as blockchain and Web3, where AI has not played a major driving role and they are not smart enough.
DeAI thus will fundamentally promote the development of intelligent decentralized technologies and systems and escalate decentralized movements, including Web3, DAOs, and DeSci.
DeAI, blockchain, DAOs, DeSci, and Web3 require and take advantage of local capabilities, resources, high computation, high bandwidth, high privacy, high security, low energy and low latency in decentralized devices and contexts. Without sharing resources, capabilities and computation with centralized servers and mediation by controlling the authority, DeAI maximizes the power of end and edge devices and resources.
Edge Intelligence
Edge intelligence emerges in synergizing edge computing with AI.
Edge intelligence refers to the intelligent paradigms, techniques, capabilities, activities, behaviors and problem-solving of end devices by those in proximity, or jointly by the end, edge, and cloud devices to achieve an end, edge (cluster), or collaborative AI.
AI goals, tasks, and capabilities are directly undertaken on decentralized end and edge devices for every device, every local system or organization, and devices in proximity or distributed to end/edge devices. Decentralized devices, end users, and their data, behaviors and capabilities carry forward AI tasks and fulfill AI problem solvers locally and in proximity.
Like CeAI, edge intelligence also fosters and fulfills general AI capabilities, such as thinking, perceiving, communicating, reasoning, planning, analyzing, learning, behaving, acting, and decision-making. Differing from CeAI, these edge intelligent capabilities are fulfilled by individual end devices (e.g., task-specific drones) or edge devices in proximity (e.g., a cluster of nearby drones).
Accordingly, edge AI studies the challenges, techniques, and opportunities of edge vision for sensing, perception, identification and recognition in the physical, virtual, or cyber-physical world; edge learning, including analytics, reasoning, inference and optimization; edge interfacing between humans/brains and devices and virtual worlds; edge conversation, including understanding and communicating in characters, voice, text, or multimedia; edge cooperation for ad hoc, on-demand or scheduled tasking physically, virtually, or dually; edge action and intervention, including response, reaction, intervention, and next-best action; and edge consensus-building, such as task orchestration, modeling, and result aggregation.
In particular, edge learning emerges as a frontier of applying AI and machine learning on devices, edge nodes and over the air. New areas of cloud edge learning, federated edge learning, mobile edge learning, and #blockchain edge learning will become paramount in 6G, smart cities, industrial IoT, unattended task under-taking and the metaverse.
Hybridizing CeAI, DAI, and DeAI
What would an AI system integrating CeAI and DeAI look like? How would synthesizing CeAI and DeAI promote AI developments? What are their major research challenges and issues? First, the integration of CeAI and DeAI is problem and requirement driven. It does not aim to enable all-round and omniscient intelligence and functionality; and also, does not incorporate and integrate all individual intelligent paradigms and objectives, such as human-level AI, social AI and natural AI into one system.
On a high level, synergizing CeAI and DeAI seek to enhance the inclusivity, complementarity, harmony, sustainability, and balance between CeAI and DeAI thinking, methodologies, functionalities and strengths. On a low level, integrative AI aims to consolidate the respective thinking, design powers, working mechanisms; functional modules of CeAI and DeAI; and to build consensus between maximizing global and local objectives simultaneously, at the same time minimizing conflicts in understanding complex systems and pursuing effective problem-solving solutions.
Harmonic, sustainable, and balanced integrative AI involves and integrates top–down and bottom–up human intelligence in mind, emotion, vision, conversation, action, and natural and social intelligence inspired by nature and society. This thinking has been widely applied in hybrid intelligent systems by integrating two-to-multiple AIparadigms, techniques, objectives, tasks, or models, from research areas, such as neural translation and ethical AI, to specific aims and tasks, such as deep Bayesian learning and explainable AI.
Second, synthesizing CeAI and DeAI can inspire new AI thinking, methodologies, research questions, and developments. New perspectives and opportunities include systematic AI thinking, inclusive AI, integrative AI systems, intelligence metasynthesis, and conflict mitigation. Each is affiliated with new research challenges and issues.