Author: Alexandre Palma
Marketing has an uncountable number of definitions, but we can define it as the activity, set of institutions, and processes for creating, communicating, delivering, and exchanging offerings that have value for customers, clients, partners, and society at large.
With the internet advance and improvement, the marketplace for consumers shifted in an extreme way. Then the market and the marketers had so much open information that was almost impossible to filter them and find interesting information.
Then Artificial Intelligence emerges, firstly with the function of helping to filter information and provide just relevant information.
As technology developed artificial intelligence has also developed, appearing new terms applied to AI such as Machine Learning, which describes the process wherein machines are given datasets and asked to “learn for themselves” to make predictions in the real-world specifically using designed algorithms. Machine Learning is considered as a subset of AI and has evolved in many ways due to the emergence of the internet and access to much larger quantities of data.
Embedded within ML is an even more specific term that is currently being used, deep learning (DL). Essentially DL is the application of ML to a complex problem.
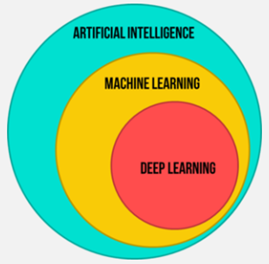
We must understand the difference between ML and predictive analysis concepts. Technically ML is a subfield of both computer science and AI, whereas a statistical model is derived from pure mathematics.
The CEO of Edvancer Eduventures, Aatash Shah clarifies that:
- ML is an algorithm that can learn from data without relying on rules-based programming.
- Statistical Modelling is a formalization of relationships between variables in the data in the form of mathematical equations.
To support and show the differences between these 2 concepts, Larry Wasserman has published in his blog a chart like this below.
Machine Learning | Statistics |
Learning | Estimation/Fitting |
Hypothesis Testing | Confirmatory Data Analysis |
Example/Instance | Data Point |
Network/Graph | Model |
Weights | Parameters |
Supervised Learning | Regression |
Unsupervised Learning | Clustering |
Feature | Covariate |
Label | Response |
Analytics Techniques
The Purpose of analytics can be either descriptive, predictive, or prescriptive.
Descriptive analytics refers to data explorations to summarize historic information.
Predictive analytics in resuming refers to methods that allow managers to forecast outcomes or answers. Is the practice of employing statistics and modelling techniques to extract information from current and historical datasets in order to predict potential future outcomes and trends.
Prescriptive Analytics is a form of advanced analytics that examines data or content to answer the question “What should be done?” or “What can we do to make?”, and is characterized by techniques such as graph analysis, simulation, complex event processing, neural networks, recommendation engines, heuristics, and machine learning.
Existing Applications of AI on Marketing
- Facebook AI is being used in marketing through targeted advertising and content curation.
- Netflix uses AI to generate recommendations for users based on their viewing history.
- Amazon uses AI to suggest other products that consumers be interested in based on purchase and search history.
These are just three of the unlimited cases in that AI is being used on Applications as a marketing tool.
But still, potential applications where AI can be applied. Advances in AI combined with changing consumer landscape have already changed the market field.
Marketing is shifting from being a conversation between two people to a conversation solely between a person and a machine. This seems like a natural progression given the current culture of turning to Siri, Alexa, or Google to answer any question or doubt.
One advantage of bots like them is that they can reduce the time between a consumer query and the answer to their questions. It is a consensus that AI will be replacing marketers, in practice, it will allow the marketing teams to skip the boring aspects and focus on the creative aspects.
While the possibility of AI seems endless, there are also real concerns to be addressed alongside the field´s advancement, concerns about the real protection of people’s private data.